PyTriton 使用教程
2024-08-15 07:28:14作者:董宙帆
项目介绍
PyTriton 是一个类似于 Flask/FastAPI 的接口,简化了 Triton 在 Python 环境中的部署。该库允许通过 NVIDIA 的 Triton Inference Server 直接从 Python 服务机器学习模型。PyTriton 支持多种操作系统,包括 Debian 11+、Rocky Linux 9+ 和 Red Hat UBI 9+。
项目快速启动
安装
PyTriton 可以通过 pip 安装,执行以下命令:
pip install nvidia-pytriton
快速启动示例
以下是一个简单的示例,展示如何在 Triton Inference Server 中运行 Python 模型:
from pytriton.decorators import batch
from pytriton.model_config import ModelConfig, Tensor
from pytriton.triton import Triton
import numpy as np
@batch
def infer_fn(**inputs):
input_data = inputs["input"]
# 模型推理逻辑
output_data = np.square(input_data)
return {"output": output_data}
with Triton() as triton:
triton.bind(
model_name="SquareModel",
infer_func=infer_fn,
inputs=[Tensor(name="input", dtype=np.float32, shape=(-1,))],
outputs=[Tensor(name="output", dtype=np.float32, shape=(-1,))],
config=ModelConfig(max_batch_size=128),
)
triton.run()
应用案例和最佳实践
应用案例
PyTriton 可以用于各种机器学习模型的部署,例如图像识别、自然语言处理等。以下是一个图像识别的示例:
from pytriton.decorators import batch
from pytriton.model_config import ModelConfig, Tensor
from pytriton.triton import Triton
import numpy as np
import cv2
@batch
def infer_fn(**inputs):
images = inputs["images"]
# 预处理图像
processed_images = [cv2.resize(img, (224, 224)) for img in images]
processed_images = np.array(processed_images)
# 模型推理逻辑
output_data = model.predict(processed_images)
return {"output": output_data}
with Triton() as triton:
triton.bind(
model_name="ImageRecognitionModel",
infer_func=infer_fn,
inputs=[Tensor(name="images", dtype=np.uint8, shape=(-1, -1, 3))],
outputs=[Tensor(name="output", dtype=np.float32, shape=(-1,))],
config=ModelConfig(max_batch_size=32),
)
triton.run()
最佳实践
- 批处理优化:使用
@batch
装饰器进行批处理,提高推理效率。 - 模型配置:合理设置
ModelConfig
中的参数,如max_batch_size
,以适应不同的推理需求。 - 错误处理:在
infer_fn
中添加错误处理逻辑,确保服务的稳定性。
典型生态项目
PyTriton 可以与其他 NVIDIA 生态项目结合使用,例如:
- NVIDIA TensorRT:用于优化深度学习模型,提高推理速度。
- NVIDIA DALI:用于数据预处理,加速数据加载和增强。
- NVIDIA Triton Inference Server:提供高性能的推理服务。
通过这些生态项目的结合,可以构建一个高效、稳定的机器学习推理服务系统。
热门项目推荐
相关项目推荐
CangjieCommunity
为仓颉编程语言开发者打造活跃、开放、高质量的社区环境Markdown00redis-sdk
仓颉语言实现的Redis客户端SDK。已适配仓颉0.53.4 Beta版本。接口设计兼容jedis接口语义,支持RESP2和RESP3协议,支持发布订阅模式,支持哨兵模式和集群模式。Cangjie032每日精选项目
🔥🔥 推荐每日行业内最新、增长最快的项目,快速了解行业最新热门项目动态~ 🔥🔥02qwerty-learner
为键盘工作者设计的单词记忆与英语肌肉记忆锻炼软件 / Words learning and English muscle memory training software designed for keyboard workersTSX022Yi-Coder
Yi Coder 编程模型,小而强大的编程助手HTML07advanced-java
Advanced-Java是一个Java进阶教程,适合用于学习Java高级特性和编程技巧。特点:内容深入、实例丰富、适合进阶学习。JavaScript085taro
开放式跨端跨框架解决方案,支持使用 React/Vue/Nerv 等框架来开发微信/京东/百度/支付宝/字节跳动/ QQ 小程序/H5/React Native 等应用。 https://taro.zone/TypeScript09Community
Cangjie-TPC(Third Party Components)仓颉编程语言三方库社区资源汇总05- Bbrew🍺 The missing package manager for macOS (or Linux)Ruby01
byzer-lang
Byzer(以前的 MLSQL):一种用于数据管道、分析和人工智能的低代码开源编程语言。Scala04
热门内容推荐
最新内容推荐
项目优选
收起
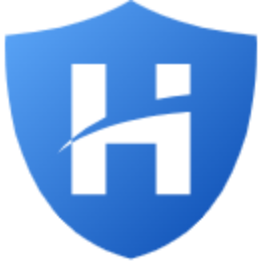
旨在打造算法先进、性能卓越、高效敏捷、安全可靠的密码套件,通过轻量级、可剪裁的软件技术架构满足各行业不同场景的多样化要求,让密码技术应用更简单,同时探索后量子等先进算法创新实践,构建密码前沿技术底座!
C
33
24
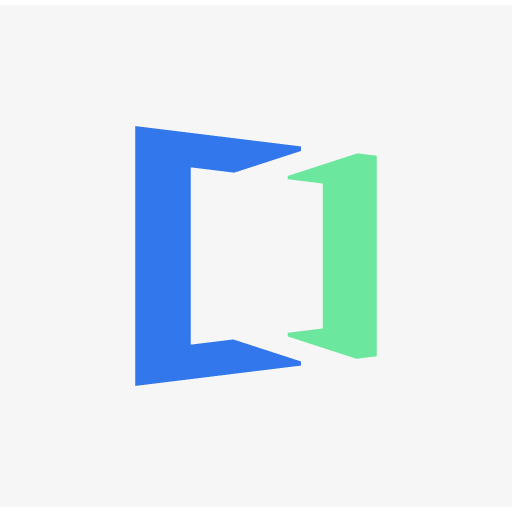
为仓颉编程语言开发者打造活跃、开放、高质量的社区环境
Markdown
828
0
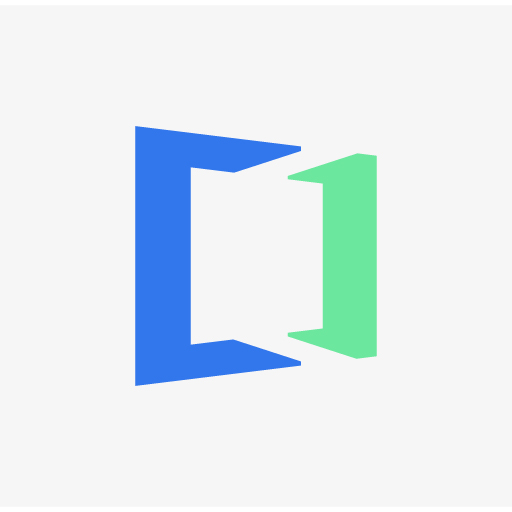
仓颉语言实现的Redis客户端SDK。已适配仓颉0.53.4 Beta版本。接口设计兼容jedis接口语义,支持RESP2和RESP3协议,支持发布订阅模式,支持哨兵模式和集群模式。
Cangjie
376
32
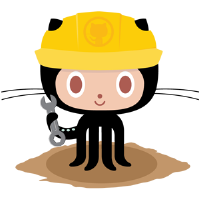
Advanced-Java是一个Java进阶教程,适合用于学习Java高级特性和编程技巧。特点:内容深入、实例丰富、适合进阶学习。
JavaScript
75.92 K
19.09 K
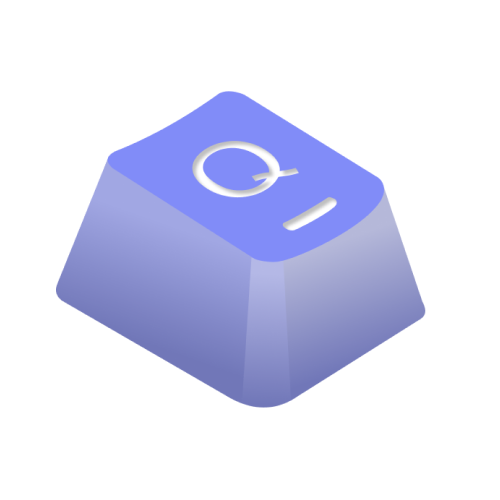
为键盘工作者设计的单词记忆与英语肌肉记忆锻炼软件 / Words learning and English muscle memory training software designed for keyboard workers
TSX
15.62 K
1.45 K
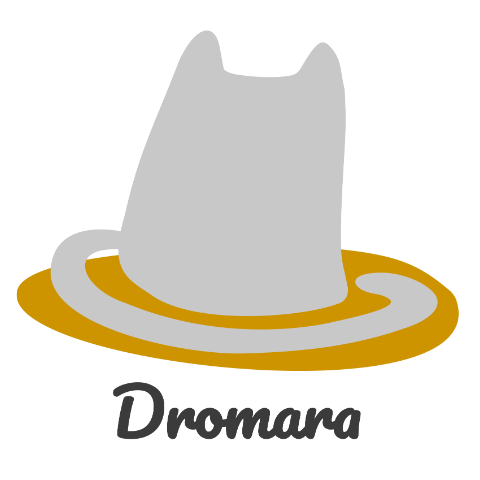
Elasticsearch
国内Top1 elasticsearch搜索引擎框架es ORM框架,索引全自动智能托管,如丝般顺滑,与Mybatis-plus一致的API,屏蔽语言差异,开发者只需要会MySQL语法即可完成对Es的相关操作,零额外学习成本.底层采用RestHighLevelClient,兼具低码,易用,易拓展等特性,支持es独有的高亮,权重,分词,Geo,嵌套,父子类型等功能...
Java
19
2
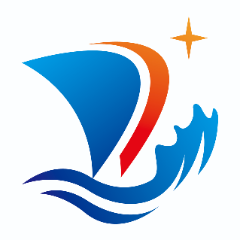
扬帆测试平台是一款高效、可靠的自动化测试平台,旨在帮助团队提升测试效率、降低测试成本。该平台包括用例管理、定时任务、执行记录等功能模块,支持多种类型的测试用例,目前支持API(http和grpc协议)、性能、CI调用等功能,并且可定制化,灵活满足不同场景的需求。 其中,支持批量执行、并发执行等高级功能。通过用例设置,可以设置用例的基本信息、运行配置、环境变量等,灵活控制用例的执行。
JavaScript
9
1
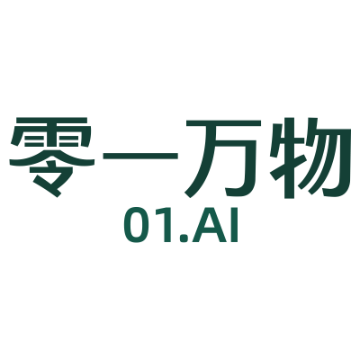
Yi Coder 编程模型,小而强大的编程助手
HTML
57
7
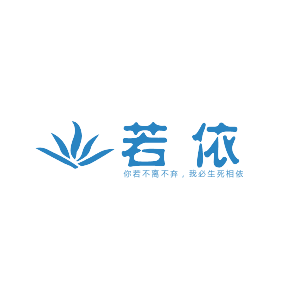
🎉 基于SpringBoot,Spring Security,JWT,Vue & Element 的前后端分离权限管理系统,同时提供了 Vue3 的版本
Java
147
26
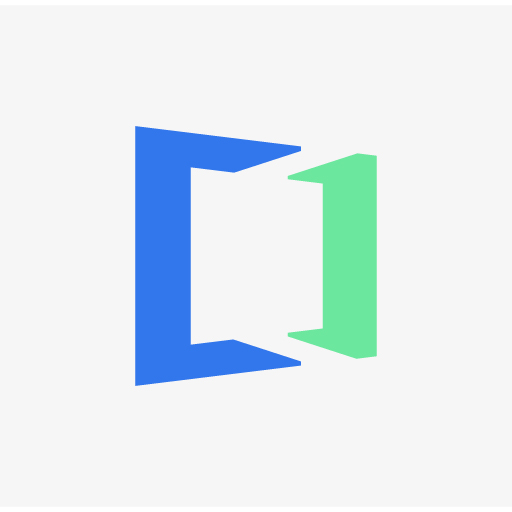
一个markdown解析和展示的库
Cangjie
10
1