TensorFlow Lite 开源项目教程
2024-08-30 19:05:43作者:温玫谨Lighthearted
项目介绍
TensorFlow Lite 是一个帮助开发者将 TensorFlow 模型转换并在移动和边缘设备上优化的工具集。目前,TensorFlow Lite 已经在超过 40 亿台设备上运行。通过 TensorFlow 2.x,开发者可以使用 tf.Keras 轻松训练模型,将其转换为 tflite 格式并进行部署,或者从模型库中下载预训练的 TensorFlow Lite 模型。
项目快速启动
安装 TensorFlow Lite
首先,确保你的开发环境已经安装了 TensorFlow。你可以通过 pip 安装:
pip install tensorflow
转换模型
以下是一个简单的示例,展示如何将一个 Keras 模型转换为 TensorFlow Lite 模型:
import tensorflow as tf
# 创建一个简单的 Keras 模型
model = tf.keras.Sequential([
tf.keras.layers.Dense(units=1, input_shape=[1])
])
model.compile(optimizer='sgd', loss='mean_squared_error')
# 生成一些示例数据
xs = np.array([-1.0, 0.0, 1.0, 2.0, 3.0, 4.0], dtype=float)
ys = np.array([-3.0, -1.0, 1.0, 3.0, 5.0, 7.0], dtype=float)
# 训练模型
model.fit(xs, ys, epochs=500)
# 转换为 TensorFlow Lite 模型
converter = tf.lite.TFLiteConverter.from_keras_model(model)
tflite_model = converter.convert()
# 保存模型
with open('model.tflite', 'wb') as f:
f.write(tflite_model)
应用案例和最佳实践
案例一:图像分类
TensorFlow Lite 广泛应用于图像分类任务。你可以使用预训练的 MobileNet 模型进行图像分类:
import tensorflow as tf
# 加载预训练的 MobileNet 模型
interpreter = tf.lite.Interpreter(model_path="mobilenet_v1_1.0_224.tflite")
interpreter.allocate_tensors()
# 获取输入和输出张量
input_details = interpreter.get_input_details()
output_details = interpreter.get_output_details()
# 进行预测
import numpy as np
from PIL import Image
img = Image.open("path_to_image.jpg").resize((224, 224))
img = np.expand_dims(img, axis=0)
img = img / 255.0
interpreter.set_tensor(input_details[0]['index'], img)
interpreter.invoke()
output_data = interpreter.get_tensor(output_details[0]['index'])
print(output_data)
案例二:语音识别
TensorFlow Lite 也适用于语音识别任务。你可以使用预训练的语音识别模型进行实时语音识别:
import tensorflow as tf
import numpy as np
# 加载预训练的语音识别模型
interpreter = tf.lite.Interpreter(model_path="speech_recognition_model.tflite")
interpreter.allocate_tensors()
# 获取输入和输出张量
input_details = interpreter.get_input_details()
output_details = interpreter.get_output_details()
# 进行预测
audio_data = np.array(..., dtype=np.float32)
interpreter.set_tensor(input_details[0]['index'], audio_data)
interpreter.invoke()
output_data = interpreter.get_tensor(output_details[0]['index'])
print(output_data)
典型生态项目
TensorFlow Lite Model Maker
TensorFlow Lite Model Maker 是一个简化自定义模型训练和转换过程的库。它提供了高级 API,使得训练和部署自定义模型变得更加容易。
pip install tflite-model-maker
TensorFlow Lite Support Library
TensorFlow Lite Support Library 提供了额外的工具和实用程序,帮助开发者更轻松地在移动和边缘设备上部署和运行
热门项目推荐
相关项目推荐
CangjieCommunity
为仓颉编程语言开发者打造活跃、开放、高质量的社区环境Markdown00redis-sdk
仓颉语言实现的Redis客户端SDK。已适配仓颉0.53.4 Beta版本。接口设计兼容jedis接口语义,支持RESP2和RESP3协议,支持发布订阅模式,支持哨兵模式和集群模式。Cangjie032每日精选项目
🔥🔥 推荐每日行业内最新、增长最快的项目,快速了解行业最新热门项目动态~ 🔥🔥02qwerty-learner
为键盘工作者设计的单词记忆与英语肌肉记忆锻炼软件 / Words learning and English muscle memory training software designed for keyboard workersTSX022Yi-Coder
Yi Coder 编程模型,小而强大的编程助手HTML07advanced-java
Advanced-Java是一个Java进阶教程,适合用于学习Java高级特性和编程技巧。特点:内容深入、实例丰富、适合进阶学习。JavaScript085taro
开放式跨端跨框架解决方案,支持使用 React/Vue/Nerv 等框架来开发微信/京东/百度/支付宝/字节跳动/ QQ 小程序/H5/React Native 等应用。 https://taro.zone/TypeScript09Community
Cangjie-TPC(Third Party Components)仓颉编程语言三方库社区资源汇总05- Bbrew🍺 The missing package manager for macOS (or Linux)Ruby01
byzer-lang
Byzer(以前的 MLSQL):一种用于数据管道、分析和人工智能的低代码开源编程语言。Scala04
热门内容推荐
最新内容推荐
项目优选
收起
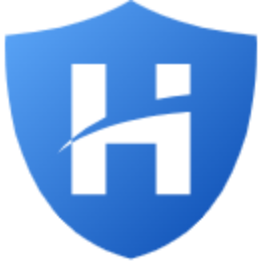
旨在打造算法先进、性能卓越、高效敏捷、安全可靠的密码套件,通过轻量级、可剪裁的软件技术架构满足各行业不同场景的多样化要求,让密码技术应用更简单,同时探索后量子等先进算法创新实践,构建密码前沿技术底座!
C
33
24
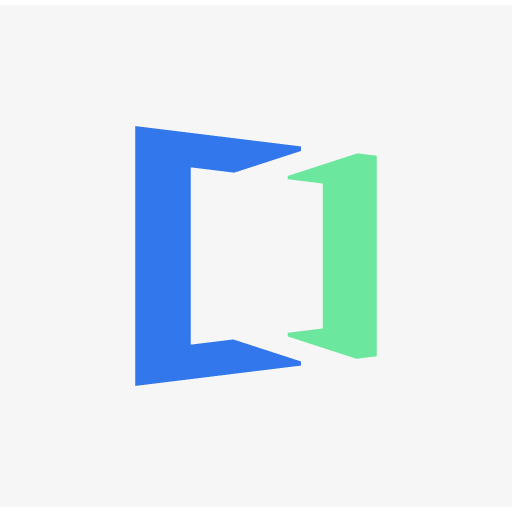
为仓颉编程语言开发者打造活跃、开放、高质量的社区环境
Markdown
825
0
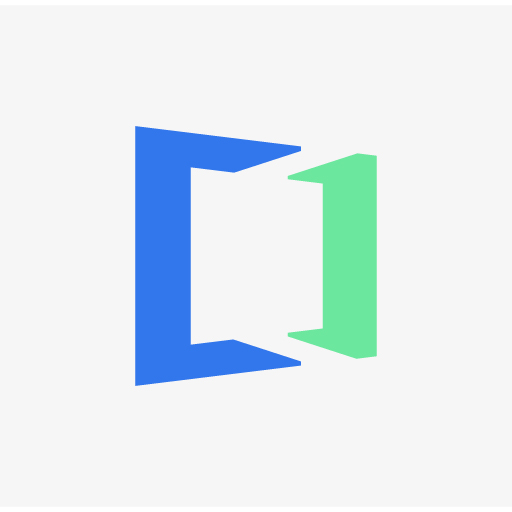
仓颉语言实现的Redis客户端SDK。已适配仓颉0.53.4 Beta版本。接口设计兼容jedis接口语义,支持RESP2和RESP3协议,支持发布订阅模式,支持哨兵模式和集群模式。
Cangjie
375
32
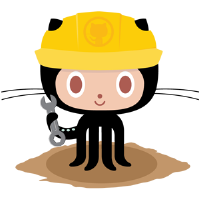
Advanced-Java是一个Java进阶教程,适合用于学习Java高级特性和编程技巧。特点:内容深入、实例丰富、适合进阶学习。
JavaScript
75.92 K
19.09 K
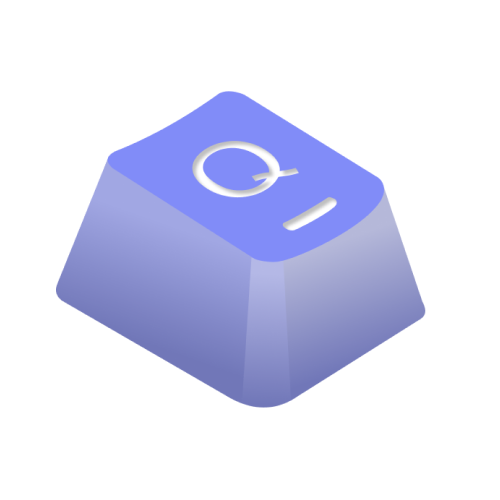
为键盘工作者设计的单词记忆与英语肌肉记忆锻炼软件 / Words learning and English muscle memory training software designed for keyboard workers
TSX
15.62 K
1.45 K
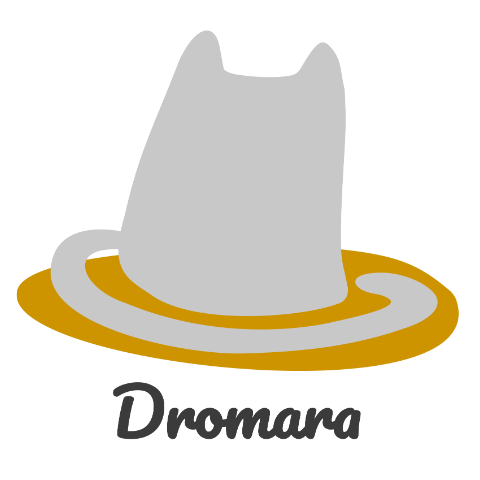
Elasticsearch
国内Top1 elasticsearch搜索引擎框架es ORM框架,索引全自动智能托管,如丝般顺滑,与Mybatis-plus一致的API,屏蔽语言差异,开发者只需要会MySQL语法即可完成对Es的相关操作,零额外学习成本.底层采用RestHighLevelClient,兼具低码,易用,易拓展等特性,支持es独有的高亮,权重,分词,Geo,嵌套,父子类型等功能...
Java
19
2
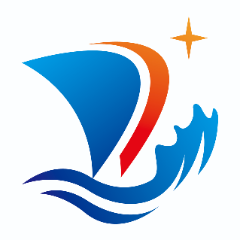
扬帆测试平台是一款高效、可靠的自动化测试平台,旨在帮助团队提升测试效率、降低测试成本。该平台包括用例管理、定时任务、执行记录等功能模块,支持多种类型的测试用例,目前支持API(http和grpc协议)、性能、CI调用等功能,并且可定制化,灵活满足不同场景的需求。 其中,支持批量执行、并发执行等高级功能。通过用例设置,可以设置用例的基本信息、运行配置、环境变量等,灵活控制用例的执行。
JavaScript
8
1
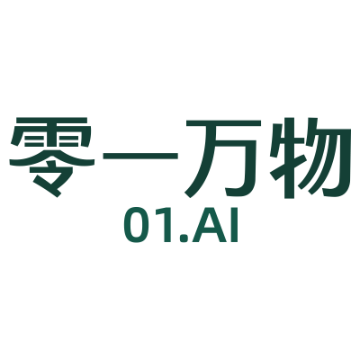
Yi Coder 编程模型,小而强大的编程助手
HTML
57
7
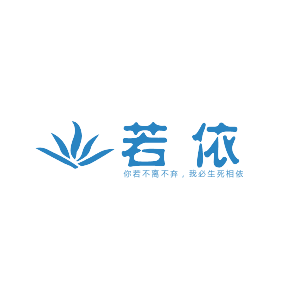
🎉 基于SpringBoot,Spring Security,JWT,Vue & Element 的前后端分离权限管理系统,同时提供了 Vue3 的版本
Java
147
26
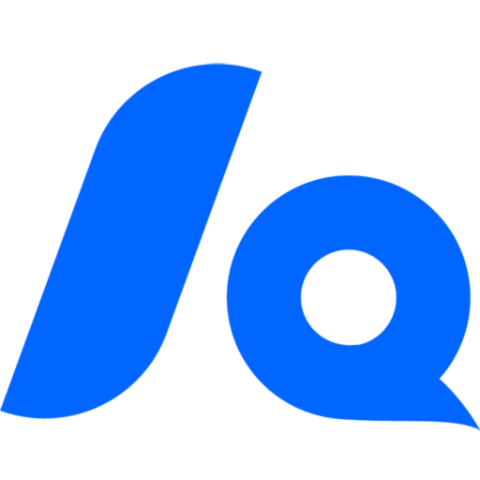
AnQiCMS 是一款基于Go语言开发,具备高安全性、高性能和易扩展性的企业级内容管理系统。它支持多站点、多语言管理,能够满足全球化跨境运营需求。AnQiCMS 提供灵活的内容发布和模板管理功能,同时,系统内置丰富的利于SEO操作的功能,帮助企业简化运营和内容管理流程。AnQiCMS 将成为您建站的理想选择,在不断变化的市场中保持竞争力。
Go
78
5